AI needs the right data
This paper seeks to ground the rush to AI in the fundamentals of setting up the right databases to support AI. AI needs relevant accurate data on which to come to relevant accurate conclusions. The adage, “Garbage in, Garbage out” may be amplified if you are unleashing the power of Artificial Intelligence. And there is a lot of garbage for AI bots to feed on.
Approximately 328.77 million terabytes of data are created each day.
176 billion terabytes of data will be generated in 2025.
We asked Chat GPT about the importance of accurate and relevant data.
ChatGPT Response:
“Accurate and relevant data is the foundation of AI's functionality. Without it, AI systems may produce inaccurate or biased results, leading to incorrect conclusions or decisions. Ensuring data quality involves various processes such as data cleaning, validation, and verification to remove errors and inconsistencies. Moreover, selecting relevant data is crucial to train AI models effectively for specific tasks. Ultimately, the quality and relevance of data significantly impact the performance and reliability of AI systems.”
Harvesting relevant Data
The data relevant to Assessors is often locked up in isolated islands of spreadsheets, word documents and private CRMs. This is the data collected by thousands of internal and external assessors seeking the truth about what organisations are actually doing. It is powerful and very relevant and needs to be unlocked.
The first step towards unleashing the power of AI for Assessment is to ensure you have the systems in place to harvest the data that Auditors are collecting every day. The data needs to be captured in databases on connected cloud systems that can be shared and analysed for effective AI.
Creating a Data Architecture for AI:
3 forms of data are important for AI:
“Causal data”. This is data on the processes, systems, capabilities and resources that make things happen the way they do. These are the opinions and evidence captured as written, audio, photo or video recordings.
“Effect data”: the outputs recorded as numbers: the number of defects, the decline in carbon emissions, the customer satisfaction scores, etc. This data can come from the assessment itself but also from data sources such as IOT, Satellites and other metric databases.
"Industry Data": such as standards, guidelines, legislation, and publications that may guide and inform.
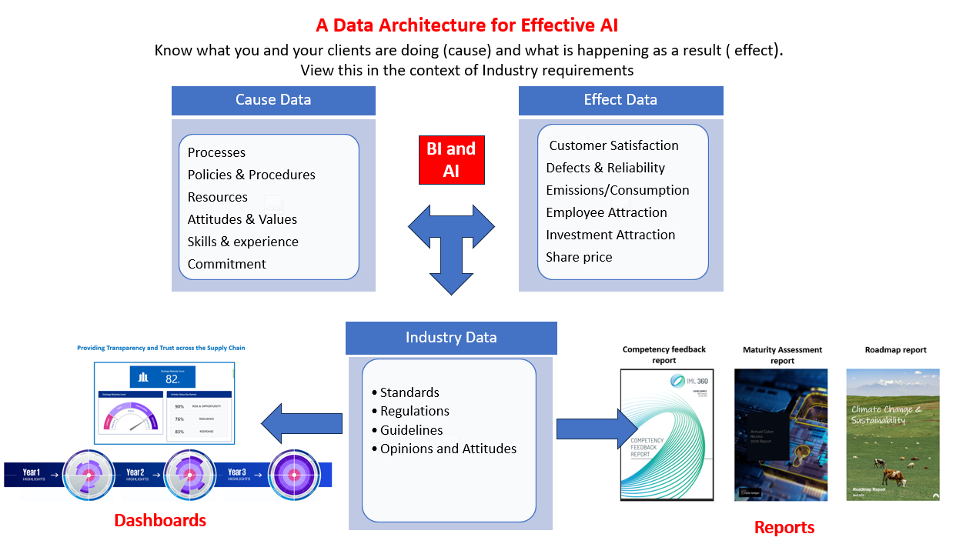
Transformational Technology:
Imagine if it was possible to share anonymous aggregated data. You could aggregate all the assessments, observations, evidence, and notes of all the internal and external auditors in your community. You could then train your AI bots to work for your auditors to produce better and more accurate reports, predict futures, develop action plans and grow their knowledge through benchmarking and cluster analysis.
Auditors would not only add greater value to their clients but could also improve the quality and consistency of their audits. With a global knowledge base and personal benchmarking to guide them auditors are more likely to come to a more consistent view no matter where they are in the world. They can also offer deeper insights and greater trust and transparency.
Ready Now:
The technology to ensure you have relevant and accessible data for AI is already here. Cloud computing and data-sharing technologies are the norm. What is needed is the will and a transformative data-driven culture. That’s the difficult part.
QuantumLeap will do the rest for you.
コメント